Comparative Study of Informative Acoustic Features for VTOL UAV Faulty Prediction Using Machine Learning
DOI:
https://doi.org/10.37934/ard.130.1.6479Keywords:
Machine learning, VTOL UAV, faulty propeller, audioAbstract
The propeller is one of the critical components in unmanned aerial vehicle (UAV) systems. The risk of the mechanism's failure could result in significant harm, hazardous events and primary maintenance services. Thus, early flying condition monitoring is necessary to ensure a stable and safe UAV operation. The sound emitted by Vertical Take Off and Landing (VTOL) UAVs offers valuable insights into their flight performance, serving as a crucial element for the efficient monitoring of flying conditions and early detection of potential faults. This paper will focus on developing fault detection and identification using audio data of different propeller conditions. The propeller faulty conditions are predicted based on informative features extracted from statistical time domain parameters of three audio wave features. Pitch, zero-crossing and short-time energy are selected as the significant audio features for the machine learning classification algorithm. UAV sounds collected in the experiment will be analysed and divided into a 60:40 ratio for training and testing datasets. Medium tree, Gaussian Naive Bayes and Ensemble Subspace k Nearest Neighbour algorithms are used for classification performance comparison. Among the three features, pitch produced the highest accuracy with 78.75% of training and 77.50% of testing using the Medium Tree algorithm.
Downloads
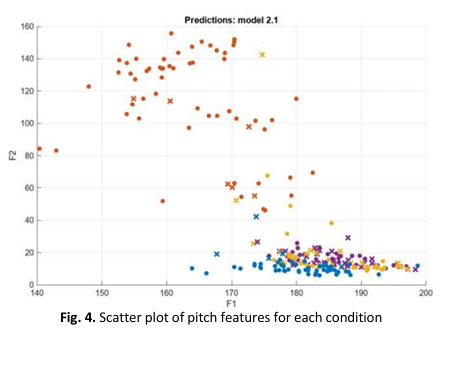