Head Tilting Angle Prediction using Machine Learning
Keywords:
Head tilting angle, machine learning , data modellingAbstract
Head tilting angle is determined to be one of the factors that leads to the occurrence of motion sickness among the vehicle driver and passengers. The mathematical model on the relationship between the effect of lateral acceleration and the motion sickness occurrence has been modelled. However, it would be complicated for the vehicle passengers and drivers to put on sensors during a journey leading to the designing of the prediction model of the head tilting angle by using machine learning. Hence, it is very important to have a system in the vehicle that is able to predict the movement behaviour of occupants particularly due to the dynamic changes of vehicle motion in which the model that produce high accuracy results that has yet been established. In order to improve the performance and reduce overfitting occurrence, the implementation of dropout layer is introduced with the variation of hidden neuron numbers in model designing. This work is conducted in order to design a prediction model for head tilting angle of car occupants using machine learning and to analyse the prediction model performance in terms of accuracy with respect to the variation of network parameters. The data modelling went through several stages for modelling and analysis purpose. The parameters are varied in terms of the number of hidden neuron that compromised the single hidden layer in the network. The modelling process are divided into passenger’s and driver’s model design without and with dropout layer implementation. The results obtained proves that the application of dropout layer in the network modelling improves the accuracy of the output for the passenger’s head tilting angle prediction response model but decreasing in the accuracy happens in driver’s head roll angle prediction response model due to the RMSE value analysed. However, the regression values for both driver and passenger’s model showed improvement in terms of regression values that proves that the implementation of dropout layer able to reduce overfitting in neural network for non-complex NN architecture.
Downloads
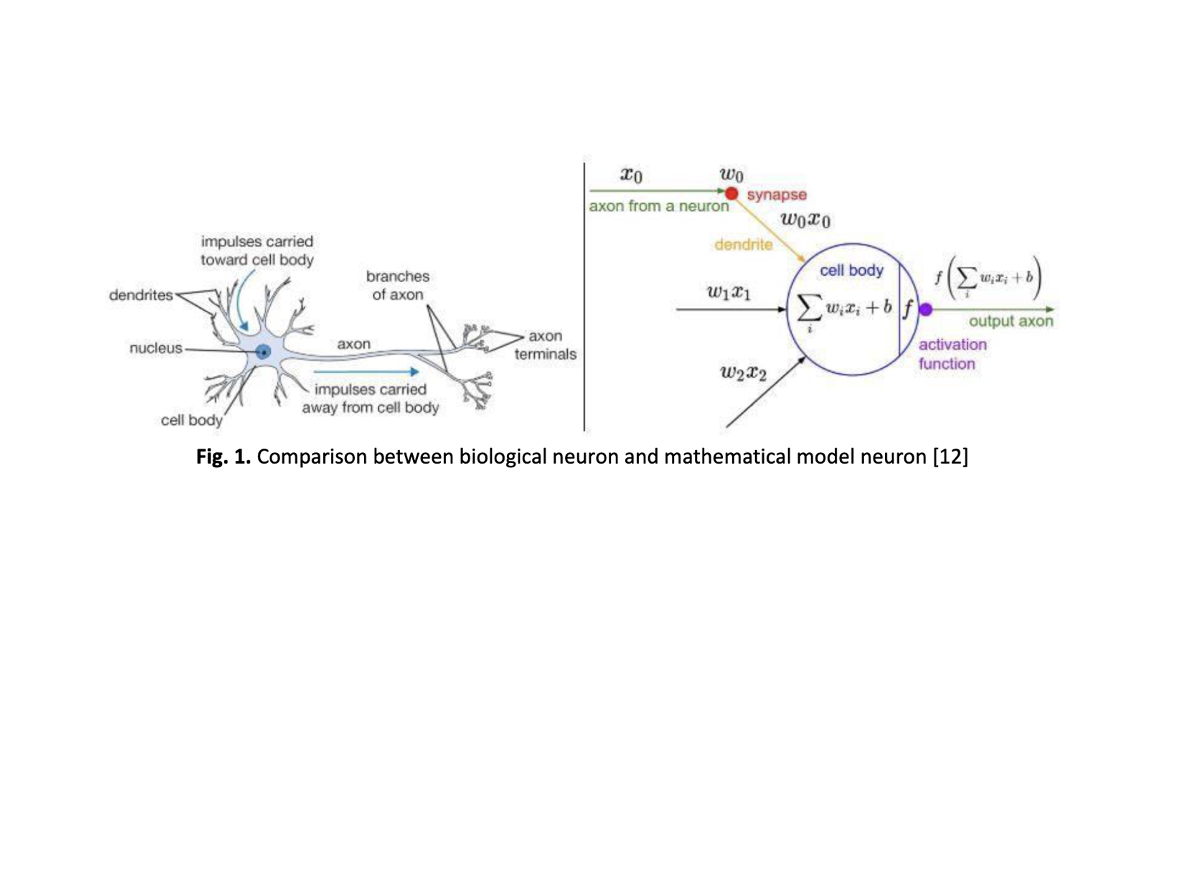