Hybrid Multilayer Perceptron Neural Network for Transformer Health Index Monitoring
DOI:
https://doi.org/10.37934/ard.129.1.89100Keywords:
transformer, dissolve gas analysis, key gas method, multilayer perceptronAbstract
Power transformers are critical to electrical systems, requiring accurate health monitoring to ensure reliability and prevent failures. Traditional assessment methods often fail to capture complex variable interactions, resulting in suboptimal maintenance strategies. This study introduces a hybrid multilayer perceptron (HMLP) neural network for transformer health index (HI) monitoring, using approximately 500 data points from the Klang Valley, including gas formation data. The HMLP is benchmarked against classifiers such as multilayer perceptron (MLP), K-Nearest Neighbors (KNN), Linear Discriminant Analysis (LDA) and Support Vector Machine (SVM), outperforming them with 92.35% accuracy and a mean squared error (MSE) of 0.78. Additionally, three training algorithms; Backpropagation (BP), Levenberg-Marquardt (LM) and Bayesian Regularization (BR) were tested, with the BR algorithm achieving the best performance at 94.13% accuracy and an MSE of 0.39. This research highlights the potential of the HMLP network, particularly when trained with BR, to revolutionize transformer maintenance by enabling precise THI predictions, facilitating proactive interventions and ensuring power system reliability.
Downloads
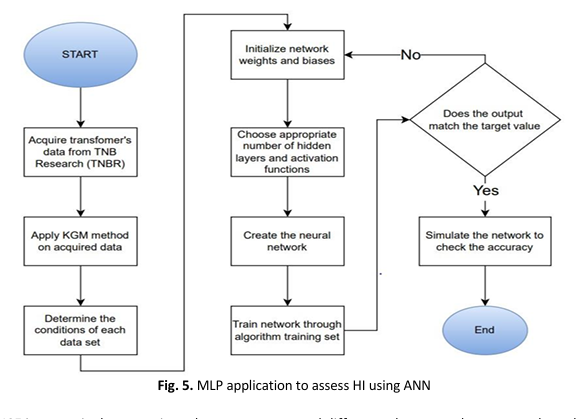