ScanNote: A Mobile Application for Enhanced Text Recognition and Digital Note-Taking Using Machine Learning-Driven Optical Character Recognition (OCR)
DOI:
https://doi.org/10.37934/ard.124.1.7789Keywords:
Mobile application, machine learning, text recognition, optical character recognition (OCR)Abstract
ScanNote, a novel note-taking application designed to address limitations in current text recognition tools. Traditional OCR systems often struggle with accurately recognizing handwritten text, rotated images or text in noisy environments, creating inefficiencies for users needing reliable digital conversion of physical documents. ScanNote integrates machine learning (ML) with OCR to enhance accuracy and adaptability, offering a solution that outperforms traditional methods. The development of ScanNote responds to the demand for a more effective tool that can seamlessly convert both printed and handwritten text into editable digital notes. Evaluation results show that for printed text, ScanNote achieves 96.3% accuracy, compared to 86.7% for traditional OCR. When text is rotated 180°, ScanNote maintains 89.7% accuracy, while traditional OCR drops to 55.3%. For handwritten text, ScanNote reaches 84.1% accuracy, outperforming traditional OCR’s 54.5%. In addition to superior text recognition, ScanNote includes core note-taking functions and export capabilities, positioning it as a competitive tool in the digital note-taking market. Future research will focus on further improving accuracy for complex texts and optimizing real-time processing. ScanNote represents a significant step forward in bridging physical and digital note-taking.
Downloads
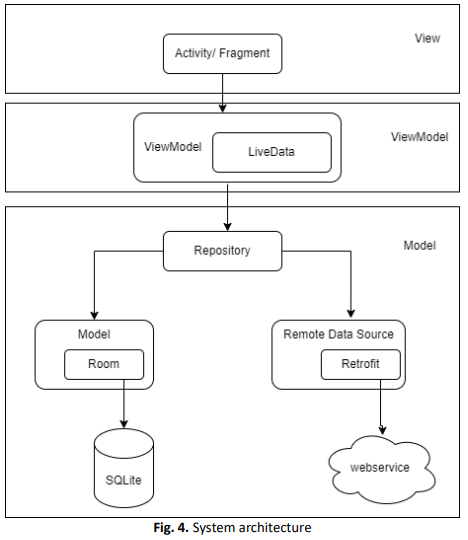