Evaluation of Machine Learning Models for Predicting Maintenance Strategies in Oil and Gas Pipelines Based on Life-cycle Cost Analysis
DOI:
https://doi.org/10.37934/ard.124.1.6376Keywords:
Machine learning models, life cycle cost analysis, predictive maintenance strategiesAbstract
The research focuses on evaluation of machine learning models in the context of predicting maintenance strategies within the oil and gas pipeline, with a primary emphasis on life-cycle cost analysis. The study underscores the crucial shift from traditional, time-based maintenance practices to data-driven, predictive maintenance strategies, which hold significant potential for enhancing safety, reliability, and cost-efficiency for pipeline operators. To address limitations associated with data availability, an innovative methodology is employed involving the generation and utilization of synthetic data. Through the simulation of diverse pipeline scenarios, the research successfully creates a comprehensive dataset for the prediction of maintenance strategies based on cost-benefit ratios. The experimental results provide valuable insights into the strengths and weaknesses of various machine learning models. Notably, Random Forest Classifier and Gradient Boosting Classifier emerge as top-performing models for classification tasks, also the predictions show that corrective maintenance has the highest frequency compared to other maintenance strategies. This study contributes significantly to the ongoing efforts to improve pipeline management within the oil and gas industry.
Downloads
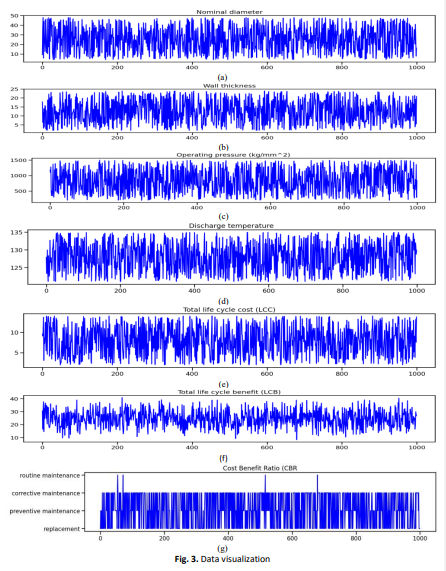