PV Fault Classification: Impact on Accuracy Performance Using Feature Extraction in Random-Forest Cross Validation Algorithm
DOI:
https://doi.org/10.37934/ard.123.1.6678Keywords:
Photovoltaic, fault classification, feature extraction, machine learning, Random-Forest, cross-validationAbstract
In light of the escalating global concerns regarding energy security and the irregular distribution of daily irradiance affecting photovoltaic (PV) system output, the demand for effective fault detection and diagnosis techniques in PV management systems is on the rise. Machine learning (ML) has emerged as the preferred approach, attracting extensive research attention. As the adoption of solar PV systems continues to surge, the need for robust fault diagnosis and classification techniques becomes paramount to ensure optimal performance, maintenance and scalability across diverse scales of PV arrays in real-world applications. This paper introduces a Solar PV Smart Fault Diagnosis and Classification (SFDC) model that harnesses the Random Forest (RF) algorithm in conjunction with Cross-Validation (CV) and an optimized feature extraction (FE) set. The deployment of CV serves to assess the model's performance and ensure its resilience. Additionally, an optimized FE set is employed to enhance classification accuracy by selecting the most pertinent features for fault classification. Scalable PV array is modelled with the generated power of 10 kW for small-scale, 250 kW for medium-scale and 2 MW for large-scale. In the training and testing of the models, the RF-CV algorithm with set combination of FE was employed to diagnose and classify different types of faults. In this process, each simulated fault that are line-line faults (LLF), open-circuit faults (OCF), ground faults (GF) and partial shading (PS) dataset was divided, with 80% allocated for training and 20% for testing purposes. As the results, all SFDC models (small, medium, large-scale) developed have achieved 100% accuracy for all fault types in training simulations. While in testing the algorithm, it effectively detected multiple fault types, especially OCF and PS, with a perfect score and slightly lower yet high accuracies for GF. These results indicate the robustness and reliability of the SFDC model in diagnosing and classifying faults in PV systems. This research not only holds the potential to advance the field of solar PV for future energy security but also serves as a valuable reference for researchers and policymakers, aiding in the optimization of PV system maintenance and operation.
Downloads
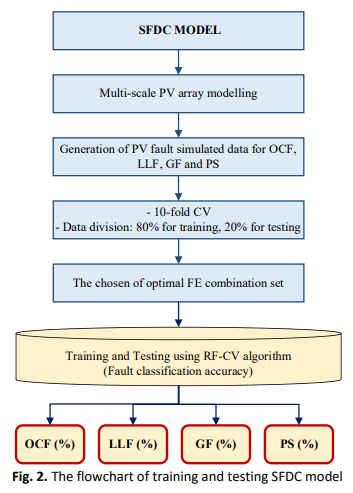