SohoNet: A Novel Social Honeynet Framework for Detecting Social Bots in Online Social Networks
DOI:
https://doi.org/10.37934/ard.122.1.234248Keywords:
Social media networks, anomaly detection, unsupervised learningAbstract
Online social networks (OSNs) are increasingly threatened by social bots – software-controlled accounts that mimic human users for various purposes. In this paper, we propose SohoNet, a novel social honeynet designed to identify, monitor, and detect these malicious entities. This innovative approach improves upon existing research by integrating multiple honeypots with a semi-automatic label engine, thereby significantly enhancing the accuracy of social bot detection. We deployed SohoNet on Platform X (formerly known as Twitter) to analyze activities during the 2022 Malaysian general election over a 14-day campaigning period. Our results show that the semi-automatic label engine successfully auto-labeled 73% of the profiles captured by SohoNet with a moderately high True Positive Rate (TPR) (0.75). Furthermore, SohoNet's overall performance (0.856), measured based on precision and capture rates, surpassed that of existing social honeypots. These findings demonstrate that SohoNet is an effective tool for detecting social bots, particularly in politically sensitive environments. However, the policy of cutting access to X API, along with the costly paid tiers introduced, poses significant challenges for future research as it restricts access to vital data and diminishes the ability to track and analyze bot behavior over time. Future work will aim to extend SohoNet's application across various OSNs to enhance its adaptability and utility.Downloads
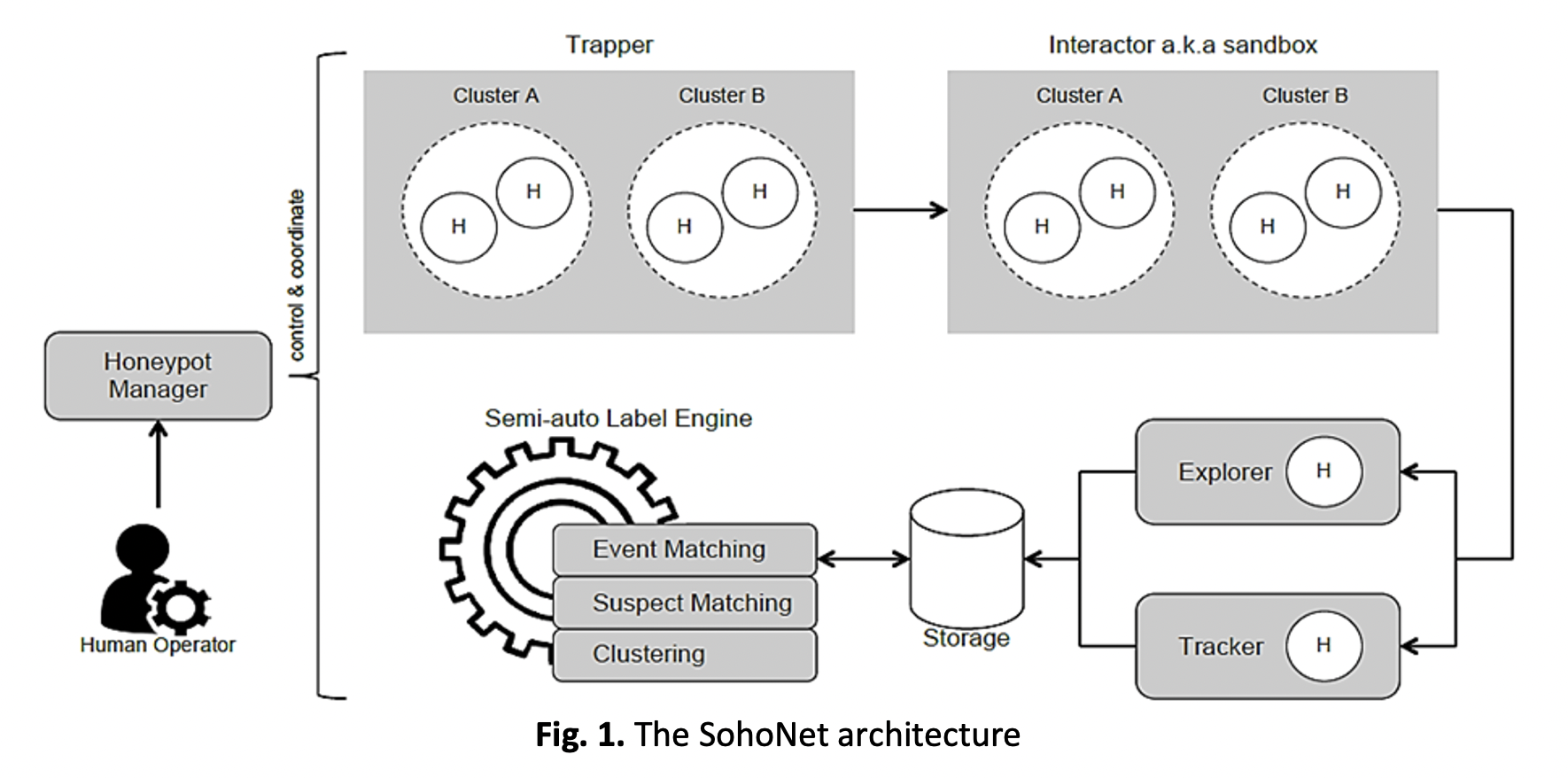