Performance Comparison of Deep Neural Networks on Lane Detection for Driving Scene
Keywords:
Deep neural network, lane detection, deep learning, autonomous vehicleAbstract
One of the main challenges in developing autonomous vehicles is lane detection. Various methods have been used for lane detection such as sensor-based, feature-based and model-based. The emergence of deep neural network approaches had shown some promising results in lane detection. In this research, 2 popular deep neural network-based models namely, Efficient Neural Network (ENet) and Efficient Residual Factorized ConvNet (ERFNet) are selected for comparative study. The selected network models were validated with the TuSimple dataset. The raw image from the dataset was pre-processed with 3 methods, they are image resizing, channel- wise normalization and random data augmentation with random image rotation by 3 degrees. Both ENet and ERFNet are trained with 50 epochs and a batch size of 20 mixed-precision are implemented. The performance of trained models are evaluated in terms of accuracy, false positive rate (FP), false negative rate (FN), number of floating-point operations performed (FLOP), parameters count and speed of network models in terms of frame per second (FPS). ENet obtained an accuracy of 95.251% under the TuSimple dataset while ERFNet obtained an accuracy of 96.035%. ERFNet having a higher number of FLOP and parameters than ENet reflects that the ERFNet requires a larger computational cost than ENet. Both ENet and ERFNet have proven to capable to operate in real-time as they were able to run in 82.75 fps and 86.16 fps respectively. Both network models were compared to the state-of-the-art method, but only ERFNet remain competitive with others as it achieves a minimum accuracy of 96%.
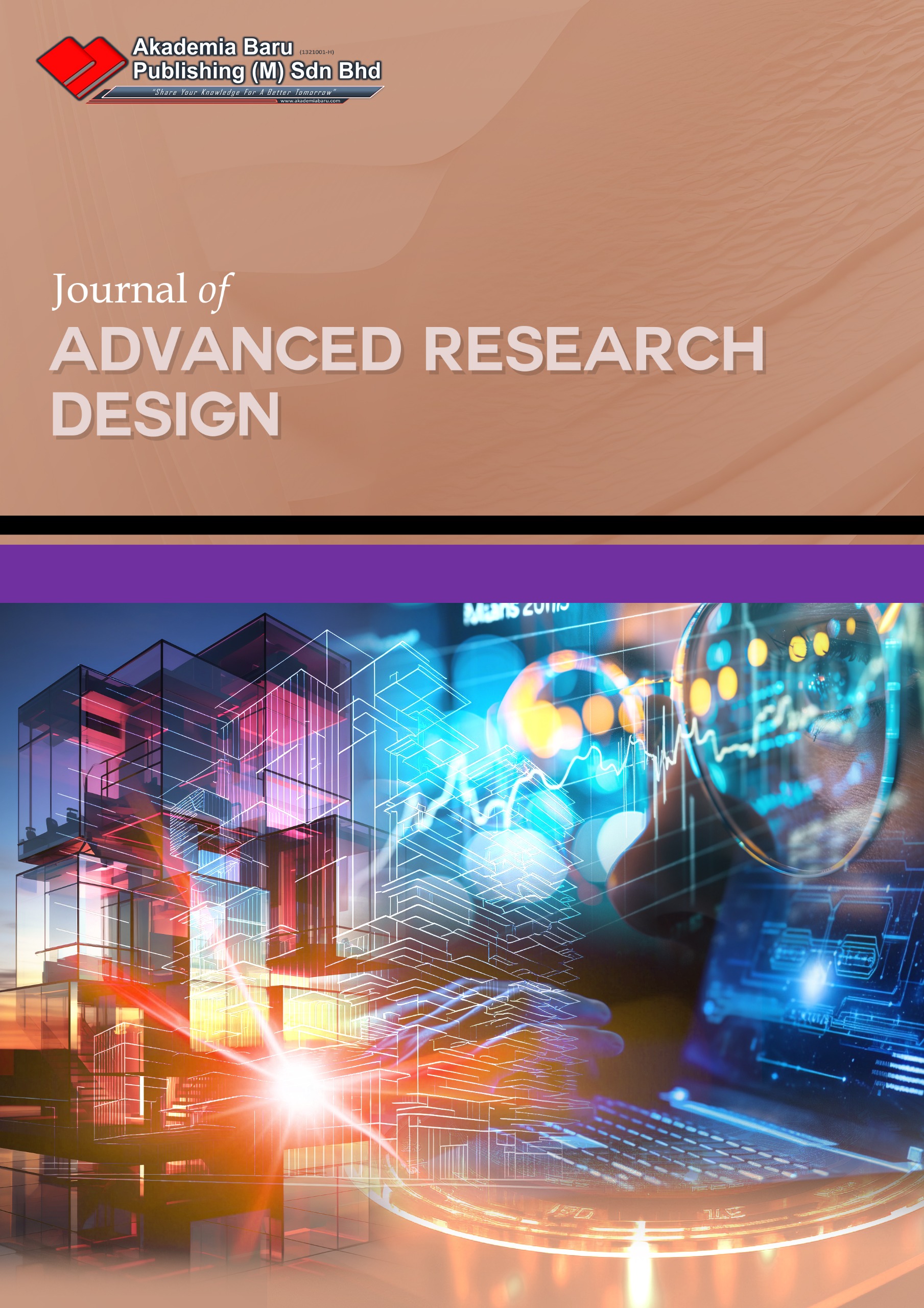