Low Power Integrated Circuit Design of Extreme Learning Machine using Power Gating Methodology
DOI:
https://doi.org/10.37934/arca.31.1.1319Keywords:
Artificial intelligence (AI), Extreme Learning Machine, Power Gating MethodologyAbstract
With the current trend of individuals seeking more advanced and intelligent devices in their daily lives, artificial intelligence (AI) chips have become the primary focus for numerous industries. To create smarter AI chips, more computing power is often required, which can lead to an increase in power consumption. This paper describes a low power design of the Extreme Learning Machine (ELM) using single voltage power gating methodology to reduce power dissipation without affecting ELM's functionality. This is realized by applying power gating and state retention techniques in logic synthesis and physical design of ELM. First, a register transfer level (RTL) ELM was designed in Verilog based on the C code of ELM. Then, its functionality was verified by the testbench using VCS. Subsequently, Design Compiler (DC) tool, which was set-up with the inclusion of libraries containing power gating components, was used to synthesize the RTL into a netlist. The hotspot module was identified by compiling the design along with testbench, and timing constraints. The hotspot is where the power gating was implemented after it was described in Unified Power Format (UPF). Then, power analysis was done in PrimeTime tool. By switching power switch on and off, the total power was decreased from 0.1127V to 0.0784V which is 30.43% reduction. In physical design of ELM, power switches are synthesized in array style. Other physical layout design flow such as floorplan, power network synthesis, placement, and clock tree synthesis (CTS) were also performed.
Downloads
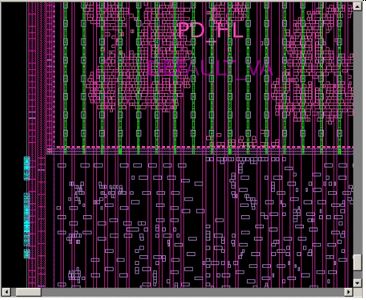