Prediction of Bubble Point Pressure for Sudan Crude Oil using Artificial Neural Network (ANN) Technique
Keywords:
Artificial Neural Network , Pressure-Volume-Temperature (PVT), Prediction of bubble point pressureAbstract
In this study, a new Artificial Neural Network predictive model was developed to determine the bubble point pressure Pb for Sudanese oil field using ANN tools in MATLAB software. Because of limitations of the experimental procedures and the time taken to obtain bubble point pressure value from the reservoir fluid samples analysis, an alternative is required where many researchers have been conducting research on the use of Artificial Neural Network (ANN) techniques. In the present study ANN model was developed and evaluated using 151 experimental data sets for Sudanese oil field, and more 61 data sets are used to compare the developed model with universal and regional published models. Comparing with universal and global empirical models, the developed model of ANN for Pb pressure has better precision index of correlation 94.63% with MSE and RMSE of 180 and 156, respectively. However, the results show that of ANN has a lower performance than regional PNN model, as the PNN shows index of correlation 97.57% with MSE and RMSE of 88 and 101, respectively. This difference may be due to the limitation in number of variables and number of data points used in each model developed. Thus, the ANN developed model in this study might be improved to predict the Pb especially for Sudan oil fields and similar oil field properties in regional by increasing the data point used in ANN model training.
References
A. Ahmed, E.-B. Ahmed, H.S. Mohamed, Development of an expert system for reservoir fluid PVT properties correlations, in: 60th Annual Southwestern Petroleum Short Course, 2013.
A. Hashemi Fath, A. Pouranfard, P. Foroughizadeh, Development of an artificial neural network model for prediction of bubble point pressure of crude oils, Petroleum. 4 (2018) 281–291. https://doi.org/10.1016/j.petlm.2018.03.009.
H. aldeen M.M. Mustafa, M.A.M. Elgasim, M.A.K. Idrees, New correlation for prediction of bubble point pressure for Sudan crude oil, 2016. http://repository.sustech.edu/handle/123456789/15198 (accessed January 12, 2021).
S.A. Nada., M.R. Iqdam, M.A. Yazan, T.A. Zainab, Development of PVT correlation for Iraqi crude oils using artificial neural network, Iraqi Journal of Chemical and Petroleum Engineering. 13 (2012) 9–16. https://ijcpe.uobaghdad.edu.iq/index.php/ijcpe/article/view/337 (accessed January 12, 2021).
S.K. Elmabrouk, Application of function approximations to reservoir engineering, 2012.
M.A. Al-Marhoun, S.S. Ali, A. Abdulraheem, S. Nizamuddin, A. Muhammadain, Prediction of bubble point pressure from composition of black oils using artificial neural network, Petroleum Science and Technology. 32 (2014) 1720–1728. https://doi.org/10.1080/10916466.2012.707267.
K.S. Arjun, K. Aneesh, Modelling studies by application of artificial neural network using matlab, Journal of Engineering Science and Technology. 10 (2015) 1477–1486.
O.B. Salem, A.G. Ahmed, B.B. Amer, Artificial intelligence techniques for predicting the reservoir fluid properties of crude oil systems, International Research Journal of Engineering and Technology. 2 (2015) 373–382.
M. Heidarian, M. Karimnezhad, M. Schaffie, M. Ranjbar, A new empirical correlation for estimating bubble point pressure using the genetic algorithm, Geology, Geophysics & Environment. 43 (2017) 33. https://doi.org/10.7494/geol.2017.43.1.33.
A. Mohamed, M. Ahmed, I. Mohamed, M. Abdein, The performance of the popular PVT correlations on Sudanese crude oils: A statistical study, in: SPE Kingdom of Saudi Arabia Annual Technical Symposium and Exhibition, Society of Petroleum Engineers, 2018. https://doi.org/10.2118/192438-MS.
S. Elkatatny, T. Moussa, A. Abdulraheem, M. Mahmoud, A self-adaptive artificial intelligence technique to predict oil pressure volume temperature properties, Energies. 11 (2018) 3490. https://doi.org/10.3390/en11123490.
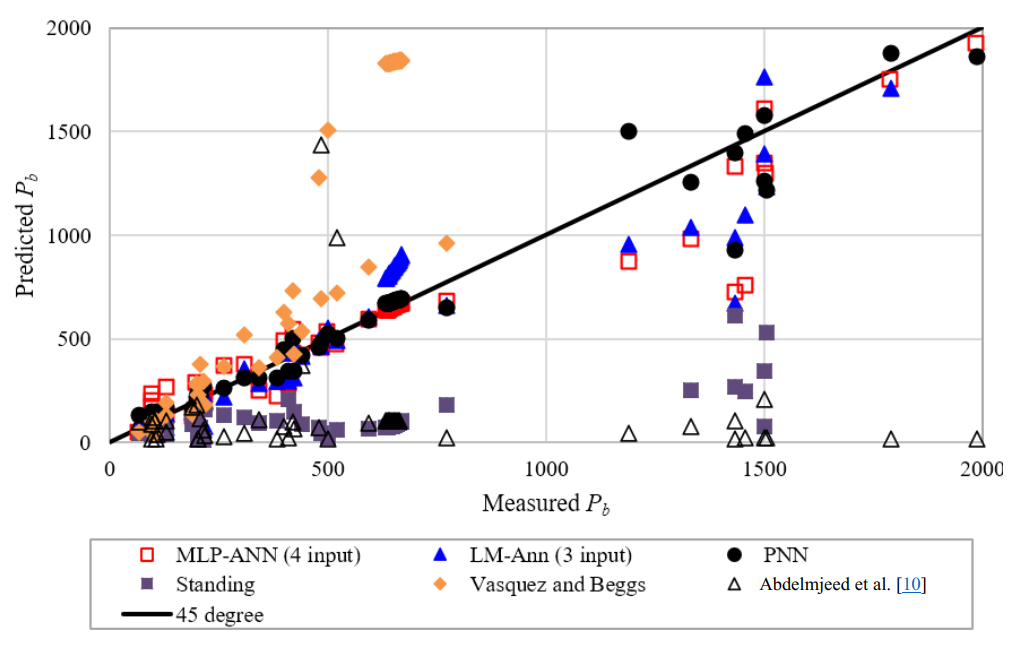
Downloads
Published
How to Cite
Issue
Section
License
Copyright (c) 2021 Progress in Energy and Environment

This work is licensed under a Creative Commons Attribution-NonCommercial 4.0 International License.