Image Detection and Distance Estimation for Rear Collision Avoidance using YOLOv4 on Jetson Nano
Keywords:
Image Detection, Distance Estimation, Rear Collision AvoidanceAbstract
Traffic accidents pose a significant global challenge, resulting in millions of non-fatal injuries annually. Addressing road safety issues and implementing comprehensive measures are crucial. This study evaluates a rear-end pre-collision avoidance system (CAS) utilizing the YOLOv4 algorithm on a small, powerful embedded computer, NVIDIA© Jetson Nano™. The system integrates image detection and distance estimation techniques within a monocular vision framework. Experimental results demonstrate the system's remarkable accuracy in distance estimation. Under normal lighting conditions, it achieved an accuracy rate of 94.60% with a confidence level of 0.778. Even in altered lighting conditions, it maintained a commendable accuracy of 94% with an increased confidence level of 0.8376. Additionally, the system effectively generated warning messages and responded when predefined distance thresholds were reached. These findings highlight the system's practical applicability, particularly in rear collision avoidance scenarios. In a broader context, this research contributes to advancing collision avoidance systems by addressing the critical need for precise distance estimation in rear-end collision scenarios. By enhancing safety and reliability, these systems have the potential to reduce accident risks and elevate road safety significantly. Future research should focus on refining the algorithm, integrating advanced technologies, and conducting extensive tests in diverse environments to optimize further and validate the system's capabilities. These efforts promise to improve road safety and extend the system's applicability in various domains.
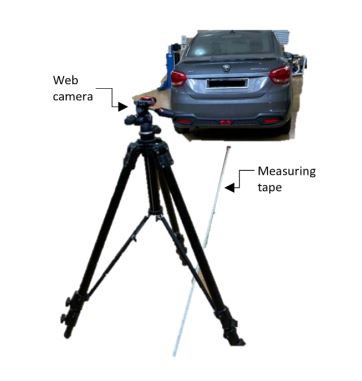