Large Scale Image Retrieval for Remote Sensing Images using Low Level Features
Keywords:
Low level features, PCA, KNN, SVMAbstract
Content Based Image Retrieval (CBIR) system extracts features relevant to query image using feature extraction method. Many low level features are proposed to retrieve accurate similar image, but the problem is no method provides accurate results. In this paper, we discuss getting an accurate result for retrieving remote sensing images from (USGS) United States Geological Survey database using different low level features. The lower level features used to construct the feature vector are Discrete Cosine Transform (DCT), Karhunen-Loève transform (KLT), Wavelet transform (WT), Histogram of orientation (HOG), and Gist. Different combinations of these features are used to train two classifier (KNN) K-nearest neighborhood and (SVM) Support Vector Machine classifier. A dimensionality reduction technique (PCA) principal component analysis is used to reduce the dimensionality of the feature vectors and see the effect of PCA on the accuracy of the classifiers.
Downloads
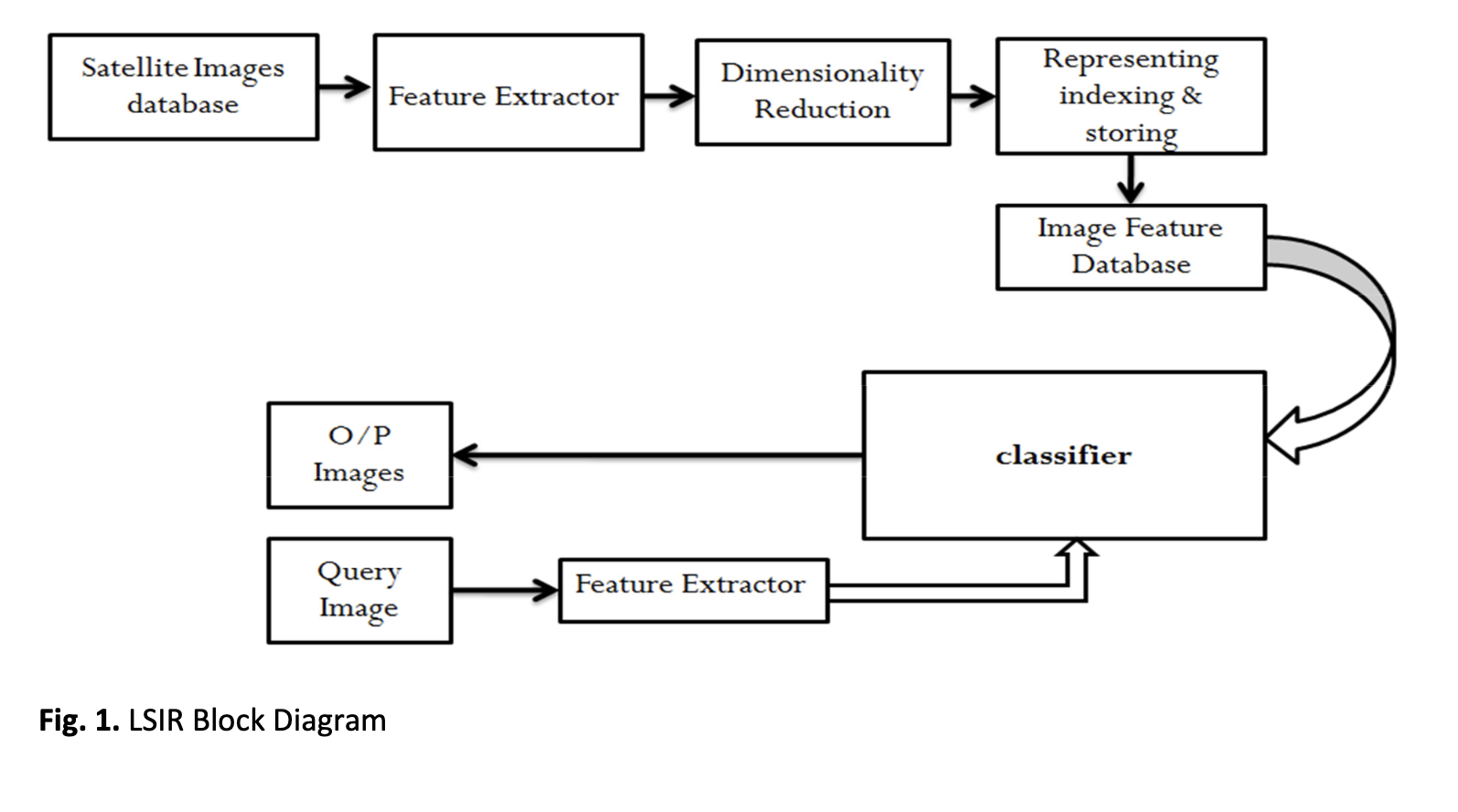